Evolutionary Therapy
Evolutionary inspired therapies are at the heart of much of our current research
The term “personalized medicine” is often applied to strategies that match tumor treatment to specific predictive biomarkers measured from an individual patient. These approaches have had some early success in prolonging patient survival time in certain cancer types. However, the reality is that the initial positive response is often followed by the evolution of drug resistance, treatment failure, and eventual patient death. Rather than ignoring this evolution, evolutionary-enlightened therapeutic approaches deliberately embrace the dynamics that govern the emergence of drug resistance and develop treatment strategies that can exploit these dynamics. Building on almost a decade of integrated research experience at the Moffitt Cancer Center in theoretical, experimental, and clinical oncology, Moffitt recently opened the Center of Excellence for Evolutionary Therapy with the intent to revolutionize personalized cancer therapy, making an individual patient’s treatment response be the driver of their own treatment schedule. One specific example of an evolutionary-enlightened therapeutic approach is Adaptive Therapy.
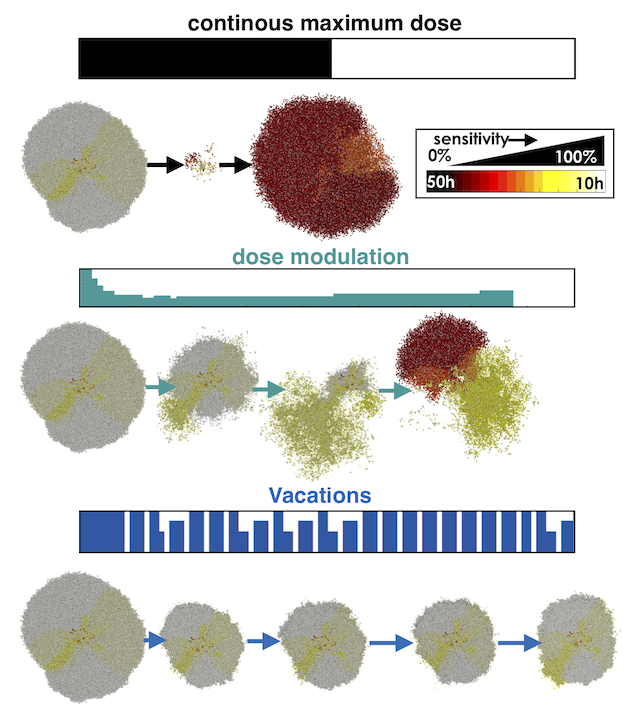
Adaptive Therapy
Many initially successful cancer therapies eventually lead to resistance and treatment failure. This is because current treatment schedules are designed for a maximum cell kill to achieve a fast cure. However, the maximum kill strategy maximally selects for drug resistant cells. Where previously their growth would have been constrained by competition from sensitive cells, they are now able to grow in an unconstrained fashion. A key research focus of our lab is to develop novel evolution-based treatment strategies that prevent, or at least delay, this competitive release and treatment failure. This so called adaptive cancer therapy modulates the drug dose according to the tumour’s growth, so to maintain a pool of sensitive cancer cells that helps to control resistance via competition. A recent clinical trial in metastatic prostate cancer here at the Moffitt Cancer Center has shown that adaptive therapy can more than double time to progression as well as reduce cumulative drug dose (see Zhang et al, 2017). Further trials are on their way in melanoma, lung, thyroid, ovarian, and breast cancer. However, important open questions remain: How do we identify patients that benefit from adaptive therapy? What is the optimal adaptive algorithm? How do we adapt treatment in light of inter- and intra-tumoral heterogeneity and distinct metastatic lesions, or multiple drugs with different targets? At the Anderson lab we are using theoretical models (ordinary differential equations, game theory, and agent-based models) combined with biological (in vitro 2D/3D culture and in vivo models), and clinical data to address these challenges.
A major challenge in adaptive therapy is how to temporally monitor cancer dynamics through time. Our research in advanced BRAF-mutant melanomas shows that the precise timing of BRAF-inhibitor withdrawal and rechallenge is critical to the success of adaptive therapy paradigms. We developed a 2-compartment ODE model that describes the competition between sensitive and resistant tumor cells and tested this on xenograft BRAF-mutant melanoma mice. Real-time model predictions of mouse-specific adaptive schedules using this model showed a reduction in burden of ~50% over continuous treatment, which motivated a phase 1 feasibility trial of adaptive intermittent BRAF-MEK inhibitor therapy in patients with advanced metastatic BRAF-mutant melanoma (NCT03543969). Model calibration resulted in a suite of parameter sets that fit the data equally well, defining a cohort of likely patient-specific responses, that predicted delayed tumor progression of up to several months if the patients were treated with adaptive therapy instead of continuous therapy (see Smalley et al, 2019).
While population dynamics models can offer insight into when adaptive therapy may be a good option for treatments, there are spatial interactions to consider as well. Dysregulated vasculature and spatial heterogeneity of tumor phenotypes can play a key role in the response of a tumor mass to a given application of therapy, particularly when treatment doses are being varied or cycled irregularly. Here, we used an off-lattice agent-based model of metastatic lesions composed of different phenotypic population mixes when exposed to an anti-proliferative drug either given continuously at the maximum tolerated dose or adaptively by dose-modulation and treatment vacations. We found that the more homogeneous, sensitive tumors are cured with continuous treatment, but even a few resistant cells will cause eventual recurrence. A steady tumor size can be maintained with adaptive therapy as long as there are sufficient sensitive cells to suppress resistant cell growth. When seeded with less heterogeneity, a dose-modulation scheme can be more effective with less dose, and when seeded with more heterogeneity, higher-dosed treatment vacations help delay recurrence. Multiple similar and dissimilar metastatic lesions treated successfully with adaptive therapy, which affects the temporal systemic tumor burden dynamics (see Gallaher et al, 2018).
Our models are often based on the assumption that there is a trade-off between proliferation and drug resistance, but this assumption has been challenged or believed to be context-dependent. In light of this, we are using a system of ODEs to explore which structural conditions are necessary to provide a benefit for adaptive therapy over standard of care. Does the tradeoff matter? How much does feedback from competition or the carrying capacity matter? And how do these elements affect treatment decisions, such as which thresholds in tumor reduction to withdraw treatment. This system of models is currently being used to make on-the-fly decisions for PARP inhibitor monotherapy in serous epithelial ovarian cancer spheroids.
Finally, we want to understand better how to adaptively administer multiple drugs. Many patients have multiple druggable targets, and different drugs can have primary and secondary roles. with the greatest efficacy and/or lowest toxicity for the primary drug, while the secondary drug is applied solely to reduce the resistant population to the primary drug. Using a game theory model, we have also investigated how to drive tumor evolution by adaptively administer multiple drugs, and keep the disease burden maintained through a series of repeatable treatment cycles (see West et al, 2019).
It is clear that many cancer therapies, while initially successful, eventually lead to resistance and failure because of the evolution of tumor heterogeneity in response to the strong selection pressures imposed by drug administration. We have shown and continue to explore via theoretical, experimental, and clinical approaches how evolutionary-based treatment strategies can improve patient outcomes. There still remains much to do, adaptive therapy is a key evolutionary therapuetic approach but there are many others. For example, sequencing treatments to exploit evolutionary double binds (see Basanta et al, 2015) or using a first strike/second strike approach to try and erradicate the disease altogether.